OWLET
Validation and technical details
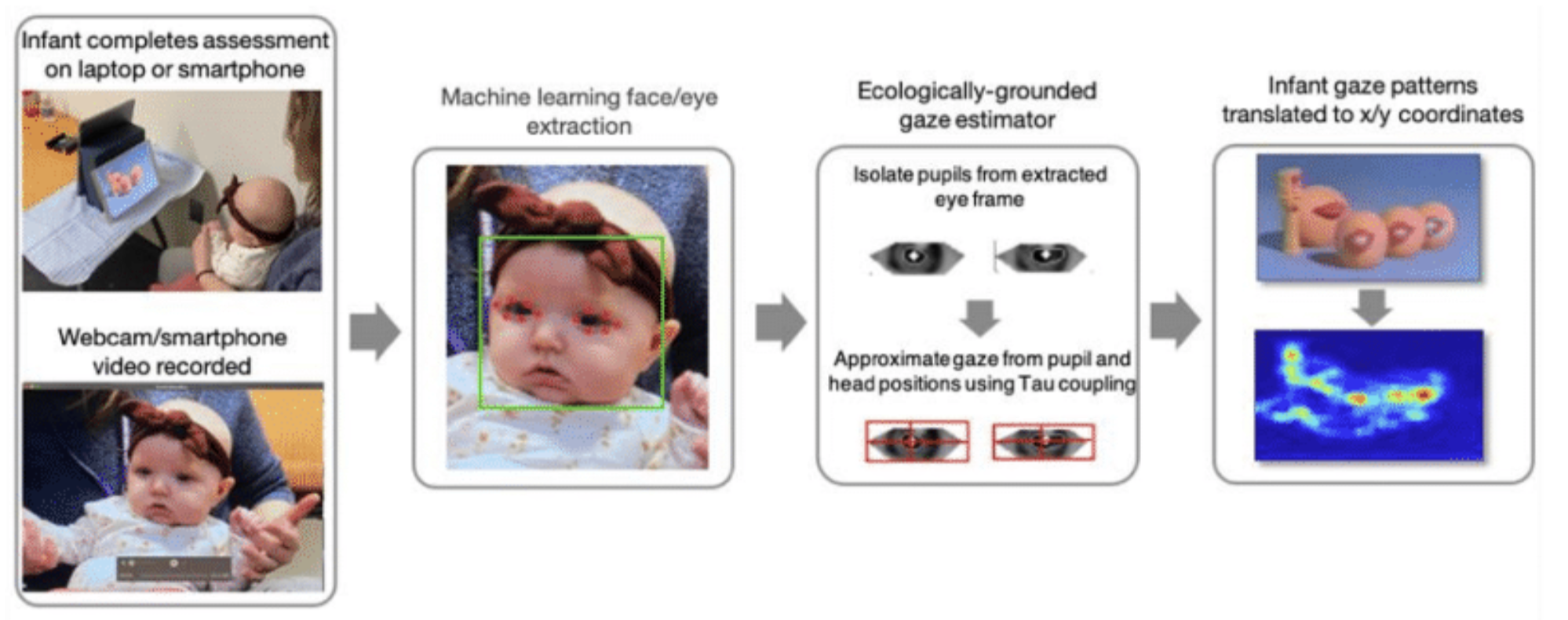
OWLET combines algorithms from computer vision, machine learning, and ecological psychology to estimate infant's point-of-gaze. I validated OWLET using data from a large sample of 6-8 month-old infants. A few key findings were:
- The average x/y spatial accuracy was 3.36°/2.67° across infants.
- There were no differences in data quality for webcams vs smartphones.
- We observed greater racial/ethnic and diversity when families were given the option to participate in remote studies using their smartphones.
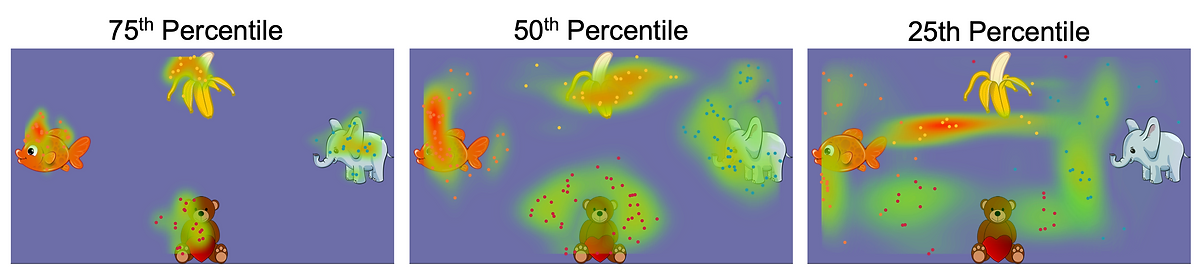
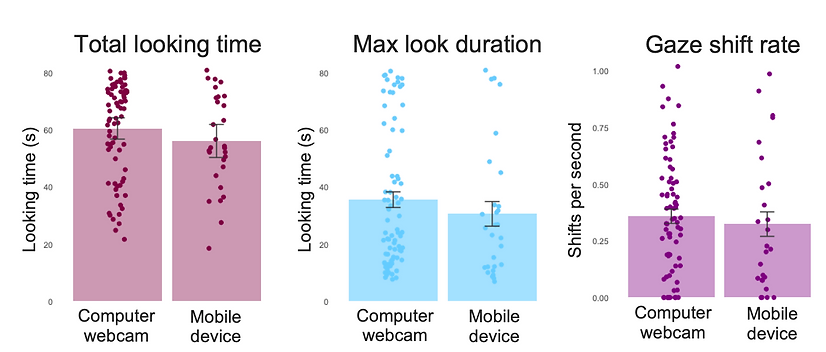
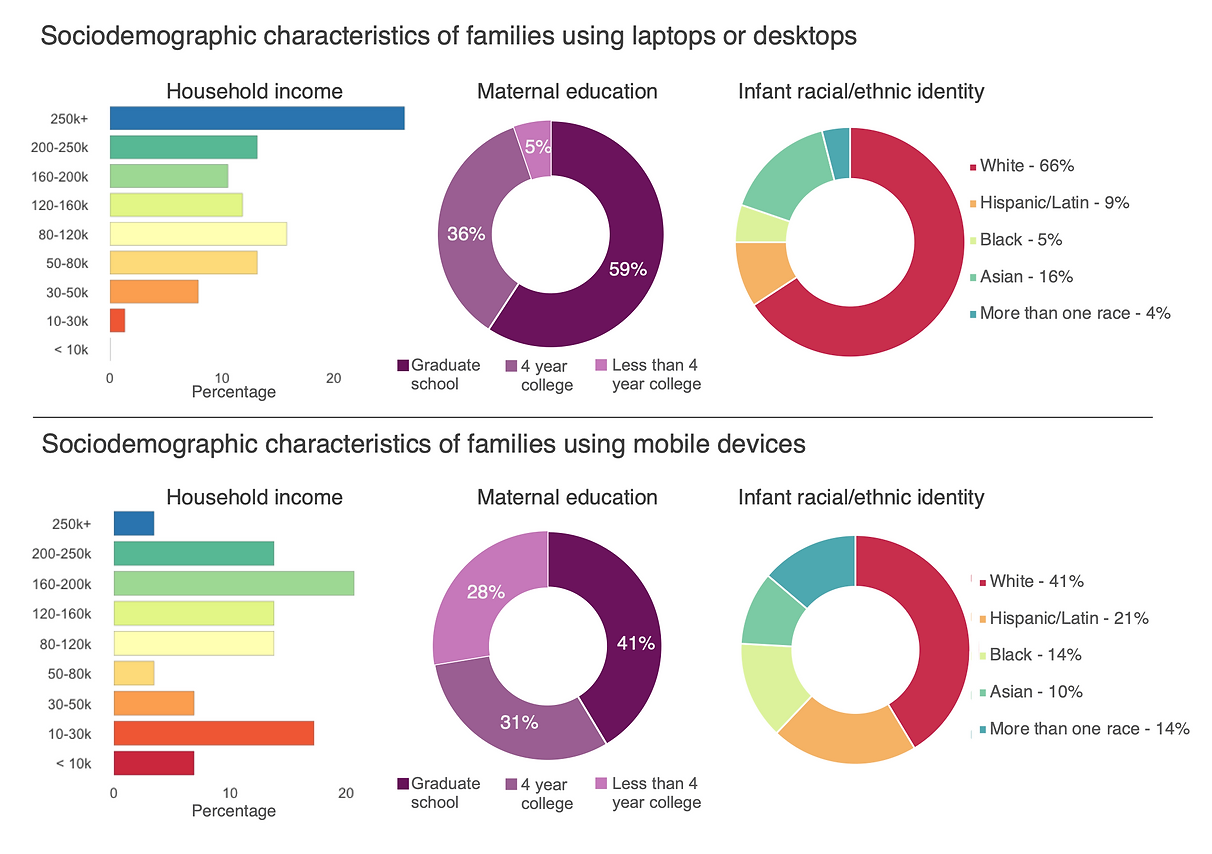
All modules, source code, and instructions for using OWLET can be found on GitHub.
Mac OSx App
I developed a beta version of a MacOSx App to better support users who are unfamiliar with using Python virtual environments. To request a password to download the app, please fill out the form below.